In today’s highly connected world, businesses and individuals alike face an increasing number of cyber threats. These threats are becoming more sophisticated, making traditional cybersecurity methods less effective in defending against attacks. Enter GAN-powered cybersecurity, an advanced approach that uses Generative Adversarial Networks (GANs) to predict and prevent cyber-attacks in real-time. This revolutionary technology leverages AI to simulate and neutralize potential threats before they cause damage, offering businesses proactive protection.
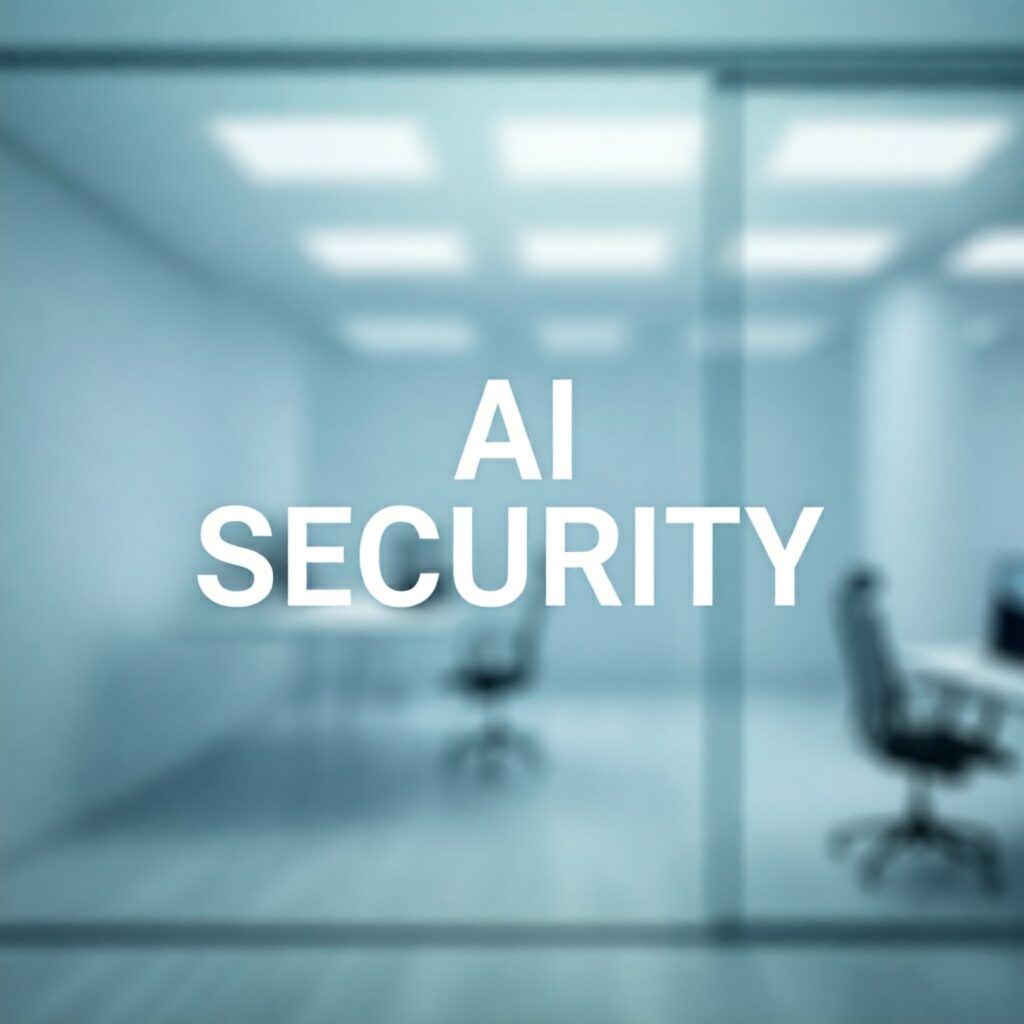
Overview of Cybersecurity Challenges Today
With the rise of digital technologies, cybercriminals have found new ways to exploit vulnerabilities across industries. Traditional cybersecurity approaches, which often rely on firewalls, antivirus software, and intrusion detection systems, are struggling to keep up with modern threats such as ransomware, phishing, and Distributed Denial of Service (DDoS) attacks. These conventional methods are inherently reactive—they only work after an attack has already occurred.
The growing sophistication of cyber-attacks, coupled with the increasing number of connected devices, has created an urgent need for more advanced, real-time defenses. Traditional methods can no longer protect organizations from zero-day attacks and novel threats. AI-driven approaches, particularly GAN-powered systems, offer a much-needed shift from reactive to proactive cybersecurity.
Why Traditional Methods Are Reactive and Limited
Most existing cybersecurity solutions operate on a reactive model. They detect threats after they have penetrated a system and often rely on pre-defined rules or known attack patterns. While this can work for known threats, it leaves systems vulnerable to zero-day attacks—new vulnerabilities that hackers can exploit before security teams can react.
Additionally, traditional defenses require constant updates to remain effective. Signature-based detection methods, for example, rely on known attack signatures and struggle against rapidly evolving threats. As a result, they are less capable of adapting to new attack methods that have not yet been cataloged.
The Emergence of AI and GANs in Cybersecurity
Artificial Intelligence (AI) has transformed cybersecurity by enabling real-time threat detection and response. Among the various AI models, Generative Adversarial Networks (GANs) are particularly promising for enhancing cybersecurity. GANs consist of two neural networks—a generator and a discriminator—that work together in an adversarial process.
- The generator creates data (e.g., simulated cyber-attacks) that it tries to pass off as legitimate.
- The discriminator evaluates the data and tries to determine whether it is real or fake.
This adversarial relationship improves both networks over time, making GANs particularly effective at predicting and defending against cyber-attacks.
What Are GANs?
A Generative Adversarial Network (GAN) is a deep learning model that trains two neural networks to work against each other to create realistic data. Essentially, GANs generate new data, such as images or music, based on an existing dataset.
In a GAN, the two networks play different roles:
- The Generator creates new data by modifying input data as much as possible to make it appear real.
- The Discriminator evaluates the generated data and tries to determine whether it’s real (from the original dataset) or fake (generated by the other network).
This process is adversarial because the two networks are in constant competition—the generator improves its outputs to fool the discriminator, while the discriminator gets better at identifying fake data. Over time, the generated data becomes so convincing that the discriminator can no longer tell the difference between the original and fake data
How GANs Work: Generator vs Discriminator
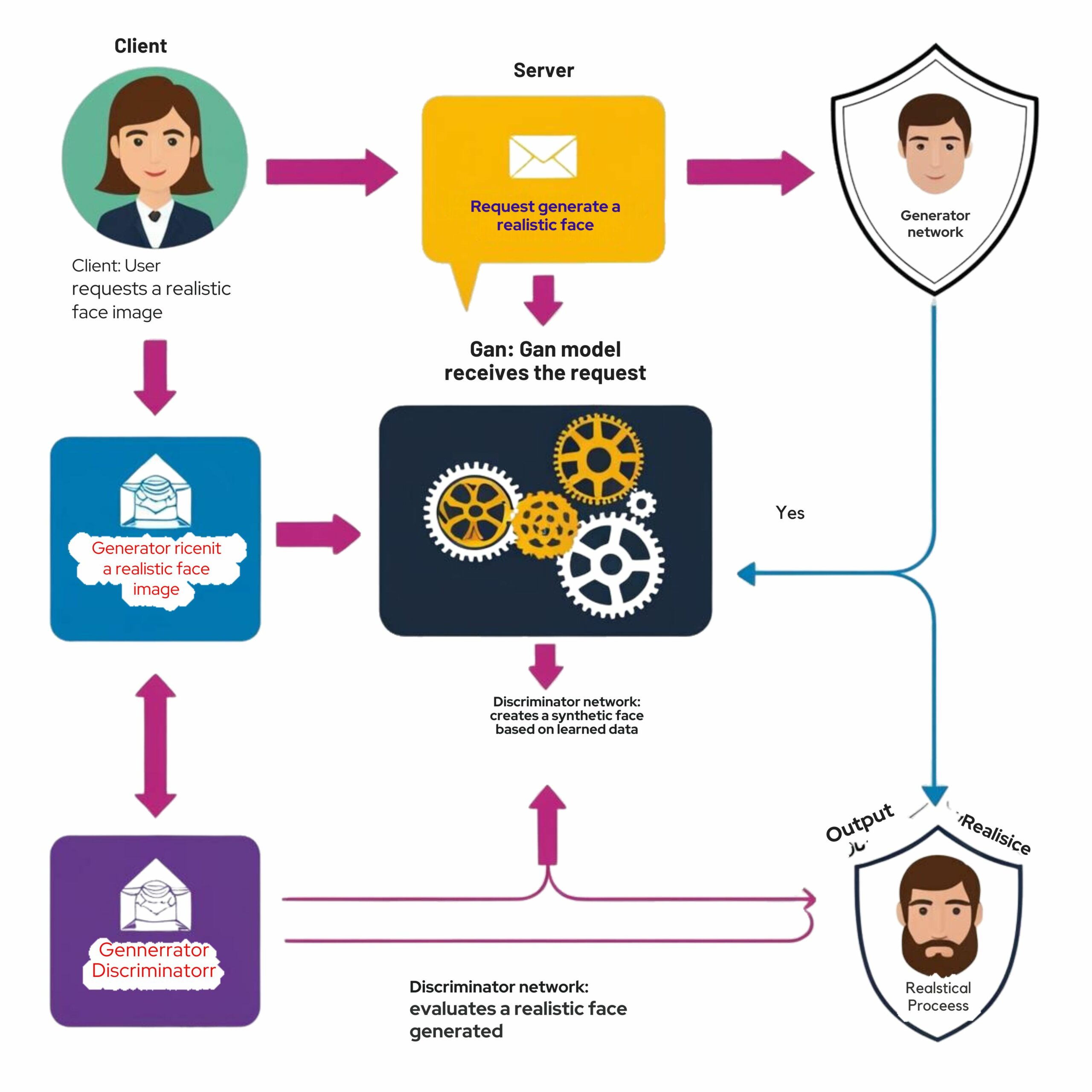
The two networks in a GAN, the generator and discriminator, play opposing roles:
Step 1: Client Request – The Client initiates a request to generate a realistic face. This request is sent as input to the server that hosts the GAN model.
Step 2: Request Forwarded – The Server receives the client’s request and forwards it to the GAN model for processing. This is where the real generation process begins.
Step 3: Generator Network – The Generator is a neural network within the GAN model. Its job is to create a synthetic face based on patterns it has learned from previous data. The generator doesn’t know if the face it creates is real or not, so it sends it to the Discriminator for evaluation.
Step 4: Discriminator Evaluation – The Discriminator is the second neural network in the GAN model. Its job is to assess whether the synthetic face generated by the generator is real or fake. The discriminator compares the generated face with real faces it has been trained on.
Step 5: Feedback Loop – If the Discriminator detects that the generated face is not realistic enough, it provides feedback to the Generator. The generator then adjusts and improves based on this feedback. This feedback loop continues until the generator creates a highly realistic face that can fool the discriminator into thinking it’s real.
Step 6: Final Output – Once the Discriminator is satisfied with the quality of the generated face, the final output is sent back to the client as a highly realistic face image.
Step 7: Client Receives the Image – The Client receives the realistic face image generated by the GAN. This completes the flow.
Application of GANs in Simulating Attack Scenarios
In cybersecurity, GANs are used to simulate various types of cyber-attacks, such as:
- Phishing attempts: GANs can generate fake phishing emails, training systems to recognize and block them.
- DDoS attacks: Simulated DDoS attacks help systems detect traffic anomalies and block malicious requests in real-time.
- Malware: GANs can mimic malware behavior, allowing security teams to detect new strains of malware before they spread.
How GANs Predict Cyber Attacks
The strength of GAN-powered cybersecurity lies in its ability to predict cyber-attacks by simulating attack patterns and leveraging real-time data. GANs can quickly analyze vast amounts of data, identify anomalies, and predict where an attack is likely to occur. This predictive ability helps organizations prevent breaches before they happen.
1.Leveraging Real-Time Data for Attack Prediction
GANs use real-time data to simulate potential attack scenarios, allowing organizations to patch vulnerabilities before they are exploited. For example, a GAN-powered system might analyze network traffic patterns to detect anomalies that could signal a forthcoming DDoS attack.
2.Training GANs to Simulate and Anticipate Threats
GANs are trained using historical data, which allows them to simulate a wide variety of attack vectors, including phishing, malware, and DDoS attacks. Once trained, GANs can anticipate new attack methods and continuously update their defenses to counter evolving threats.
GANs in Proactive Cyber Defense
Most cybersecurity systems are reactive, meaning they respond to threats only after they have occurred. GAN-powered cybersecurity flips this model by offering a proactive approach. Instead of waiting for an attack, GANs simulate potential threats and neutralize them before they can infiltrate a system.
1.Shift from Reactive to Proactive Cybersecurity
With GANs, organizations no longer have to react to attacks after they’ve occurred. Instead, they can anticipate and neutralize threats in real-time, minimizing damage and reducing the risk of breaches.
2.Real-Time Threat Detection and Neutralization
GAN-powered systems excel at detecting and neutralizing threats in real-time. By continuously simulating new attack vectors, these systems can prevent breaches before they happen. This real-time defense is especially critical in industries where data breaches could have catastrophic consequences, such as healthcare and finance.
Next Step with us
GAN-powered cybersecurity offers a revolutionary approach to protecting against cyber-attacks. By predicting and simulating attack scenarios, GANs enable organizations to anticipate and neutralize threats before they occur. This proactive, real-time defense is critical for industries like healthcare and finance, where data breaches can have devastating consequences. As AI technology continues to advance, GAN-powered systems will play an increasingly vital role in keeping businesses safe from the ever-evolving threat of cyber-attacks.
The future of GAN-powered cybersecurity is crucial for defending against cyber-attacks in real-time and Hospitals can rely on GAN-powered cybersecurity to protect sensitive patient data.”